We have prepared several datasets that we have used in several of our projects and research experiments. Please refer to this data set page and the specific publications to acknowledge the data source:
HUMAN ACTION UNDERSTANDING
- Human-to-Robot Handovers of Cups with Water
- Human Manipulation of Cups with Water
- Gaze Behavior in Dyadic Interaction
- Ball Placing and Giving
- BoxED: Box packing with Everyday items Dataset
CAMERA CALIBRATION
VIDEO SURVEILLANCE AND HUMAN HEALTH MONITORING
- A Kinect Dataset from Therapies with ASD Adults
- AHA-3D: A Labelled Dataset for Senior Fitness Exercise Recognition and Segmentation from 3D Skeletal Data
- HDA Dataset: High Definition Analytics Camera Network for surveillance
- SEAGULL Dataset: Multi-camera multi-spectrum ocean images from a UAV
- MarSyn Dataset
- KS20 VisLab Multi-View Kinect skeleton dataset
- Improved annotation for the INRIA person data set
MISC: CAMERA EVALUATION, 3D MODELLING, ROBOTIC PARTS
Human-to-robot Handovers of Cups with Water
This experiment is presented as a collaborative task, where the human should help the robot clean the table by handing over the cups, from the rightmost cup to the leftmost, one at a time. The robot receives the cup (see Figure 2a); in case the cup contains water, it pours the content into the orange bucket (Figure 2b), and finally, it places the empty cup in the blue drawer (see Figure 2c).
BoxED: Box packing with Everyday items Dataset
September 2022
Download link: .git
BoxED is the first publicly available collection of human experts packing groceries into a box. The dataset was collected in Virtual Reality (VR) and captures many parameters of the box packing task, including 6-DOF pick-and-place grasp poses, object trajectories, packing sequence and more. BoxED enables learning models for multiple aspects of this task from humans, that generate human-like behaviors.
Human Manipulation of Cups with Water
July 2021. Related project: ACTICIPATE. Download link: .zip (21 GB)
Human-Human Interaction scenario where a cup with different water levels (empty, half-full, and full) are manipulated to perform two types of actions: handover and pick-and-place. This dataset is composed of data from two sensors mounted on the two humans participating in the experiment: head-mounted eye-tracker and infra-red markers for motion capture. The dataset provides video, eye, and gaze information from the PupilLabs system, and 3-D position and 4-D quaternion information of the wrist and head of the two humans in the whole experiment.
If you use this dataset in your work, please cite the following publication(s):
- Robot Learning Physical Object Properties from Human Visual Cues: A novel approach to infer the fullness level in containers: N. F. Duarte, M. Raković and J. Santos-Victor, 2022 International Conference on Robotics and Automation Paper page
Gaze Behavior in Dyadic Interaction
April 2018. Related project: ACTICIPATE. Download link: zip (15.78 GB), md5 checksum.
The Gaze Behavior in Dyadic Interaction Dataset has videos of egocentric view with gaze visualization, raw gaze data and labeled type of action paired for two actors performing intrapersonal (placing) and interpersonal (giving) action. For further information, please contact rakovicm at uns dot ac dot rs.
If you use this dataset in your work, please cite one of the following publications:
- A dataset of head and eye gaze during dyadic interaction task for modeling robot gaze behavior, M. Raković, N. Duarte, J. Tasevski, J. Santos-Victor, B. Borovac, International Conference on Electromechanics and Robotics (ER(ZR) 2018). St. Petersburg, Russia, 18-21 April 2018. PDF file
- The Gaze Dialogue Model: Nonverbal Communication in Human-Human and Human-Robot Interaction, M. Raković*, N. Duarte*, J. Marques, A. Billard, and J. Santos-Victor, IEEE Transactions on Cybernetics (2022). PDF file *equal contribution
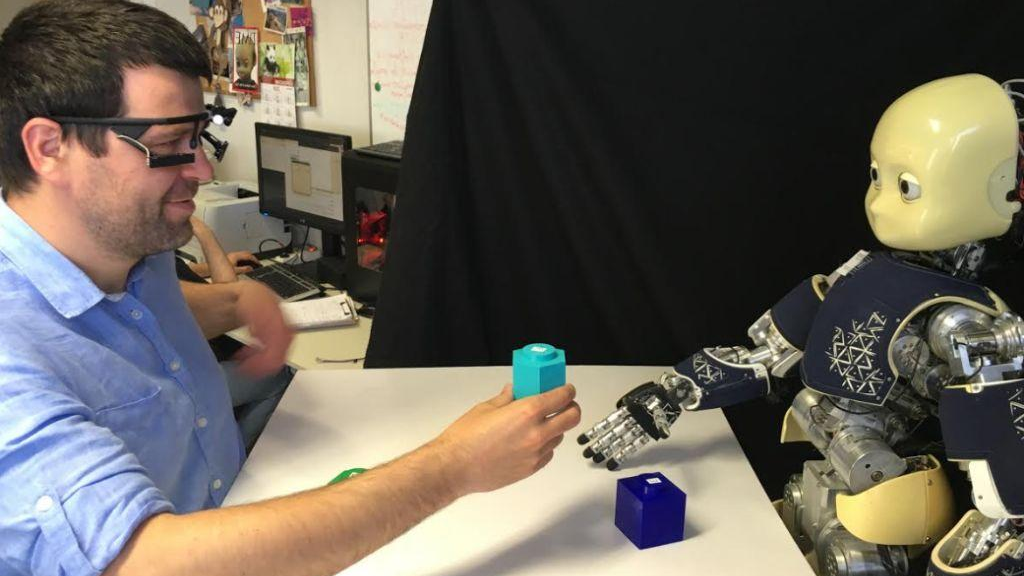
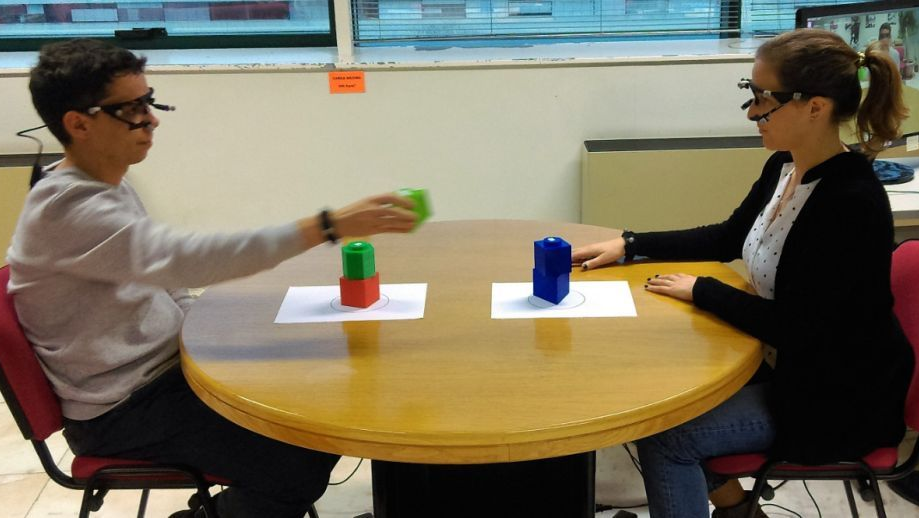
Ball Placing and Giving
September 2017. Related project: ACTICIPATE. Download link: zip (1.4 GB), md5 checksum, description of the questionnaires of the human studies.
The Ball Placing and Giving Dataset has synchronised motion capture and gaze data with labeled video of actor performing ball placing and giving actions. For further information, please contact rakovicm at uns dot ac dot rs.
If you use this dataset in your work, please cite the following publication(s):
- Anticipation in Human-Robot Cooperation: A Recurrent Neural Network Approach for Multiple Action Sequences Prediction, P. Schydlo, M. Raković, L. Jamone and J. Santos-Victor, IEEE International Conference on Robotics and Automation (ICRA). Brisbane, Australia, 21-25 May 2018. PDF file
- Action Anticipation: Reading the Intentions of Humans and Robots, N. Duarte, M. Raković, J. Tasevski, M. Coco, A. Billard and J. Santos-Victor, IEEE Robotics and Automation Letters (RA-L), July 2018. PDF file, journal page
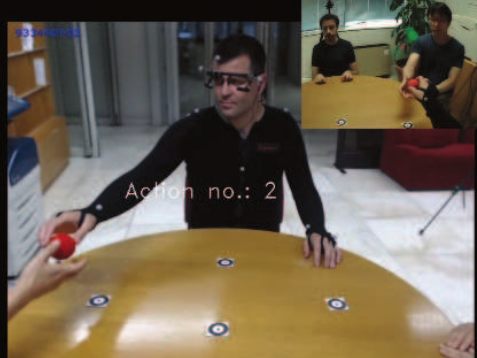
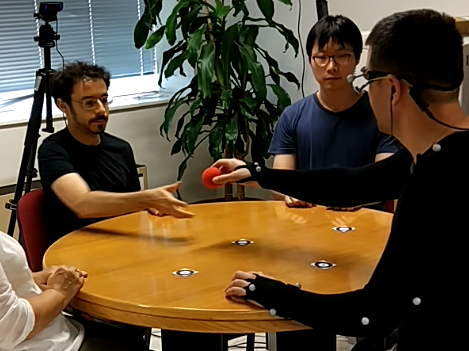
A Kinect Dataset from Therapies with ASD Adults,
April 2021.
This dataset is constituted by acquisitions done with Microsoft Kinect v2 during triadic robotic therapies for adults with Autism Spectrum disorder. It is constituted by several mirroring exercises, in which both a therapist and the patient participated. These exercises were conceived for the training gestural capabilities. The data provided is of 3-D skeletal data from videos, as well as skeleton joints in 2D color frame and the exercises executed.
If you use this dataset in your work, please cite the following publication(s):
- One-shot action recognition in challenging therapy scenarios, A. Sabater, L. Santos, J. Santos-Victor, A. Bernardino, L. Montesano, A. Murillo, Workshop on Learning from Limited and Imperfect Data (L2ID), IEEE/CVF Conference on Computer Vision and Pattern Recognition Workshops.
- Interactive social games with a social robot (IOGIOCO): Communicative gestures training for preschooler children with autism spectrum disorder, L. Santos, S. Annunziata, A. Geminiani, E. Brazzoli, A. Caglio, J. Santos-Victor, A. Pedrocchi, and I. Olivieri, I Congresso Annuale Rete IRCCCS Neuroscienze e Neuroriabilitazione, 2020.
- Available on this repository
AHA-3D: A Labelled Dataset for Senior Fitness Exercise Recognition and Segmentation from 3D Skeletal Data,
September 2018. Related project:AHA.
The AHA 3-D dataset shows both young and elder subjects performing standard fitness exercises designed for evaluating the overall fitness level in elders. These exercises are essential for estimating factors like risk of falling or overall autonomy of the subjects performing the exercises. The data provided is of 3-D skeletal data from videos, as well as frame-level labels (i.e.: one label per frame). Below we show two sample images, one depicting the Unipedal Stance exercise, and one depicting the 8-Feet Up and Go exercise.
If you use this dataset in your work, please cite the following publication(s):
- AHA-3D: A Labelled Dataset for Senior Fitness Exercise Recognition and Segmentation from 3D Skeletal Data,J. Antunes, A. Bernardino, A. Smailagic, D. Siewiorek, Vision for Interaction and Behaviour Understanding (VIBE) Workshop, British Machine Vision Conference (BMVC). Newcastle upon Tyne, UK, 3-6 September 2018. PDF file
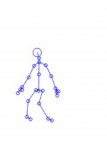
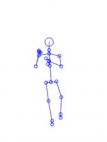
HDA Dataset: High Definition Analytics Camera Network at ISR,
July 2013. Dataset page. Related project: AHA.
HDA is a multi-camera high-resolution image sequence dataset for research on high-definition surveillance. 18 cameras (including VGA, HD and Full HD resolution) were recorded simultaneously during 30 minutes in a typical indoor office scenario at a busy hour (lunch time) involving more than 80 persons. Each frame is labeled with Bounding Boxes tightly adjusted to the visible body of the persons, the unique identification of each person, and flag bits indicating occlusion and crowd.
The following figures show examples of labeled frames: (a) an unoccluded person; (b) two occluded people; (c) a crowd with three people in front.
If you use this dataset in your work, please cite the following publication(s):
- The HDA+ data set for research on fully automated re-identification systems, D. Figueira, M. Taiana, A. Nambiar, J. C. Nascimento, A. Bernardino, Workshop, European Conference on Computer Vision (ECCV), 2014. PDF
SEAGULL Dataset: Multi-camera multi-spectrum Ocean images from UAV point of view,
2013 – 2015. Dataset page. Related project: SEAGULL.
A multi-camera multi-spectrum (visible, infra-red, near infra-red and hyperspectral) image sequences dataset for research on sea monitoring and surveillance. The image sequences are recorded from the point of view of a fixed wing unmanned aerial vehicle (UAV) flying above the Atlantic Ocean and over boats, life rafts and other objects, as well as over fish oil spills.
If you use this dataset in your work, please cite the following publication(s):
- A Dataset for Airborne Maritime Surveillance Environments, R. Ribeiro, G. Cruz, J. Matos, A. Bernardino, IEEE Transactions on Circuits and Systems for Video Technology, November 2017. PDF
MarSyn Dataset
2022, Dataset page
The Maritime Synthetic (MarSyn) dataset consists of 25 different photo-realistic video sequences, containing each one 1000 frames, obtained using Blender. Its 25 thousand images and their corresponding 34 thousand annotations aim to simulate multiple maritime scenarios and conditions such as sunny, cloudy and sunset environments, near coast images and reflections on the water. Different types of vessels are deployed in the simulations, which increases the diversity of the dataset
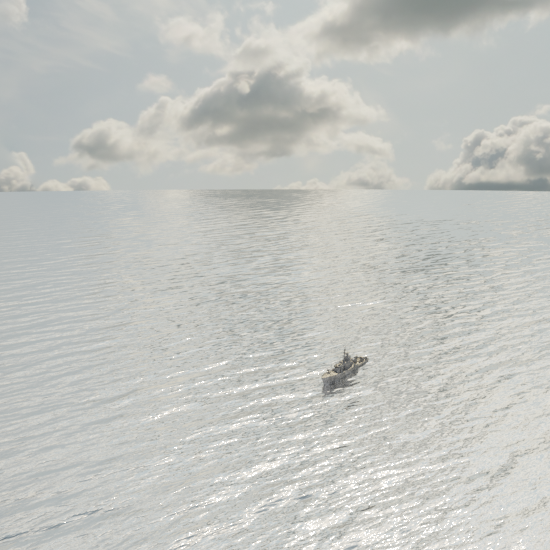
KS20 VisLab Multi-View Kinect skeleton dataset,
Athira Nambiar, 2017. Related project: AHA.
The KS20 VisLab Multi-View Kinect skeleton dataset is a set of Kinect skeleton (KS) data sequences comprised of 300 skeletal gait samples, collected from 20 walking subjects, in the context of long-term person re-identification using biometrics. The dataset is composed of the skeleton sequences of 20 subjects walking along 5 different view-points (0o, 30o, 90o, 130o, 180o) collected in-house using Kinect v2.
If you use this dataset in your work, please cite the following publication(s):
- Context-Aware Person Re-identification in the Wild via fusion of Gait and Anthropometric features, A. Nambiar, A. Bernardino, J. C. Nascimento and A. Fred, B-WILD Workshop at 12th IEEE International Conference on Automatic Face & Gesture Recognition (FG). Washington DC, USA, 30 May – 3 June 2017. PDF
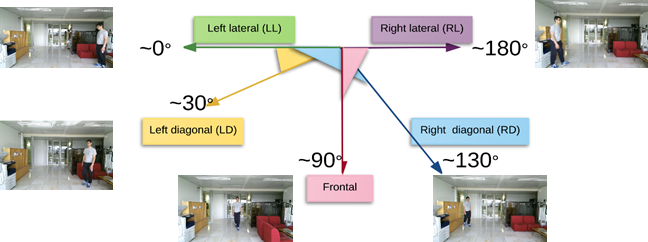

Improved annotation for the INRIA person data set,
Matteo Taiana, 2014
The INRIA person data set is very popular in the Pedestrian Detection community, both for training detectors and reporting results. Yet, its labelling has some limitations: some of the pedestrians are not labelled, there is no specific label for the ambiguous cases and the information on the visibility ratio of each person is missing. We collected a new labelling that overcomes such limitations and can be used to evaluate the performance of detection algorithms in a more truthful way. It also allows researchers to test the influence of partial occlusion and pedestrian height during training training on the detection performance. The labels are encoded in the format used for the Caltech Pedestrian Detection Benchmark, in two vbb files.
Related papers:
- On the purity of training and testing data for learning: the case of Pedestrian Detection, M. Taiana, J. C. Nascimento and A. Bernardino, Neurocomputing, vol. 150, part A, February 2015. PDF
- An Improved Labelling for the INRIA Person Data Set for Pedestrian Detection, M. Taiana, J. C. Nascimento, A. Bernardino, Iberian Conference on Pattern Recognition and Image Analysis (IbPRIA), 2013. PDF, video talk
Lytro Depth Dataset: Lytro Depth Range Assessment Dataset for 1st Generation Lytro,
January 2018.
The Lytro Depth Dataset consists of seven sub-datasets acquired with different zoom and focus settings for a 1st generation Lytro camera. These sub-datasets encompass images for calibration and depth range assessment. The object used for depth range assessment was placed parallel to the encasing of the camera and at a regular spacing of 0.05 m from the camera for depth values ranging from 0.05 to 2.00 m. The Lytro Depth Dataset has a total of 245 poses for calibration and 480 poses for depth range assessment.
The following figure shows examples of a viewpoint image with the grid used for depth range assessment at different depths.
Download links:
http://dx.doi.org/10.17632/n379z9dmpg.1 (part 1), http://dx.doi.org/10.17632/df5yynk7yn.1 (part 2)
If you use this dataset in your work, please cite the following publication(s):
- Depth Range Accuracy for Plenoptic Cameras, N. B. Monteiro, S. Marto, J. P. Barreto and J. Gaspar, Computer Vision and Image Understanding (CVIU), vol. 168C, pp. 104-117, January 2018. PDF file, supplementary material
Real-World Data for 3D Reconstruction Algorithms: Tracked features in uncalibrated images,
Etienne Grossmann, July 1999
- Complete dataset, including all the images, compressed tar file (~6MB)
- Light version of the dataset, comprising a selection of the images, compressed tar file (~700KB)
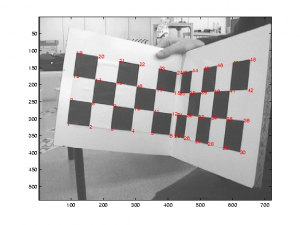
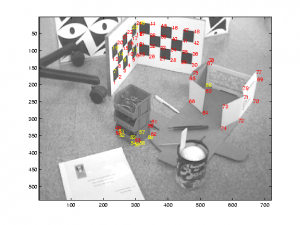
Hand Posture Affordances Dataset with the iCub,
September 2017.
This dataset contains results of trials in which the robot executes different actions with multiple hand postures on various objects. In the image we show the experimental setup, with the iCub humanoid robot at the beginning of a robot–object interaction trial, and the visual perception routines in the background screen. For more information, please contact gsaponaro at isr dot tecnico dot ulisboa dot pt.
If you use this dataset in your work, please cite the following publication(s):
- Learning at the Ends: From Hand to Tool Affordances in Humanoid Robots, G. Saponaro, P. Vicente, A. Dehban, L. Jamone, A. Bernardino, J. Santos-Victor, IEEE International Conference on Developmental and Learning and on Epigenetic Robotics (ICDL-EpiRob). Lisbon, Portugal, 18-21 September 2017. PDF
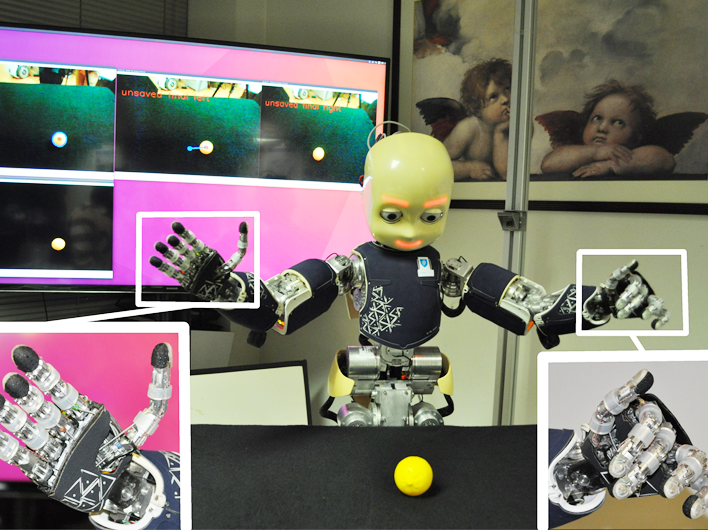
Available on this GitHub repository.
Visual Affordances of Objects and Tools Dataset,
September 2016.
This dataset contains results of trials in which the iCub humanoid robot executes different motor actions with multiple tools onto various objects. In total, there are 11 objects, 4 actions, 3 tools and at least 10 repetitions of each trial, which sums up to ~1320 unique trials and 5280 unique images of resolution 320×200 pixels. The images are captured from left and right cameras of the robot before and after executing a successful action. We also provide the foreground segmented images of each trial. Moreover, the 3D position of the object together with some extracted features are also available. For more information, please contact adehban at isr dot tecnico dot ulisboa dot pt.
If you use this dataset in your work, please cite the following publication(s):
- A Moderately Large Size Dataset to Learn Visual Affordances of Objects and Tools Using iCub Humanoid Robot, A. Dehban, L. Jamone, A. R. Kampff, J. Santos-Victor. European Conference on Computer Vision (ECCV), Workshop on Action and Anticipation for Visual Learning, Amsterdam, The Netherlands, 8-16 October 2016. PDF
Available on this GitHub repository.
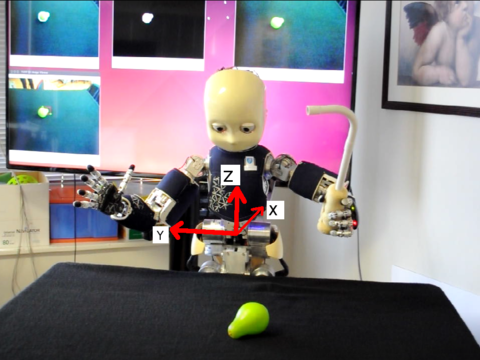
Robot Table Cleaning datasets:
1. “iCub, clean the table!” A robot learning from a demonstration approach using Deep Neural Networks,
April 2018.
In this work, the iCub robot will automatically clean a table from different types of dirt (scribbles, lentils). In order to create the cleaning trajectory, the system needs three reference frames (2D rotation matrix (A) and origin (b) expressed in the iCub reference frame (x, y, 0)). The information of the reference frames is automatically extracted from robot camera images, using a deep neural network previously trained on images taken during human demonstrations (kinesthetic teaching) of a cleaning task.
The dataset for the network is composed by 1000 cleaning demonstrations (500 for wiping off scribbles and 500 for sweeping the lentils). Technical details are given in the file README.txt. For more information, please contact j.kim at sssup dot it.
Download link: zip (182MB)
If you use this dataset in your work, please cite the following publication(s):
- “iCub, clean the table!” A robot learning from demonstration approach using Deep Neural Networks, J. Kim, N. Cauli, P. Vicente, B. Damas, F. Cavallo, J. Santos-Victor, IEEE International Conference on Autonomous Robot Systems and Competitions (ICARSC). Torres Vedras, Portugal, 25-27 April 2018. PDF file Best paper award.
2. Robot Table Cleaning v2,
July 2018.
This is a second dataset with images and right hand trajectory recorded during a kinesthetic demonstration of a cleaning task to the iCub robot. Two different cleaning motions (sweeping and wiping) were shown to the robot in order to clean two different types of dirt (scribbles and lentils). The dataset images are a projection of the robot camera images to a virtual camera plane placed on top of the table (for a detailed information on the virtual camera configuration and placement please refer to [1] and [2]). The trajectories are expressed as 200 x and y table plane coordinates.
The dataset contains 3 different sub-datasets. The first one are the original 659 kinesthetic demonstrations recorded on the iCub robot. The second one is the augmentation (13839 elements) of the original dataset used in the ICDL-EpiRob paper [1]. The third one is the augmentation (13839 elements) of the original dataset used in the JINT paper [2].
Technical details are given in the file README.txt. For more information, please contact .
Download link: zip (1.1 GB), md5.
If you use this dataset in your work, please cite the following publication(s):
- [1] Autonomous table-cleaning from kinesthetic demonstrations using deep learning, N. Cauli, P. Vicente, J. Kim, B. Damas, A. Bernardino, F. Cavallo, J. Santos-Victor, IEEE International Conference on Developmental and Learning and on Epigenetic Robotics (ICDL-EpiRob). Tokyo, Japan, 16-20 September 2018. PDF File
- [2] Cleaning tasks knowledge transfer between heterogeneous robots: a deep learning approach, J. Kim, N. Cauli, P. Vicente, B. Damas, A. Bernardino, J. Santos-Victor, F. Cavallo, Journal of Intelligent and Robotic Systems (submitted), 2018. Pre-Print Article
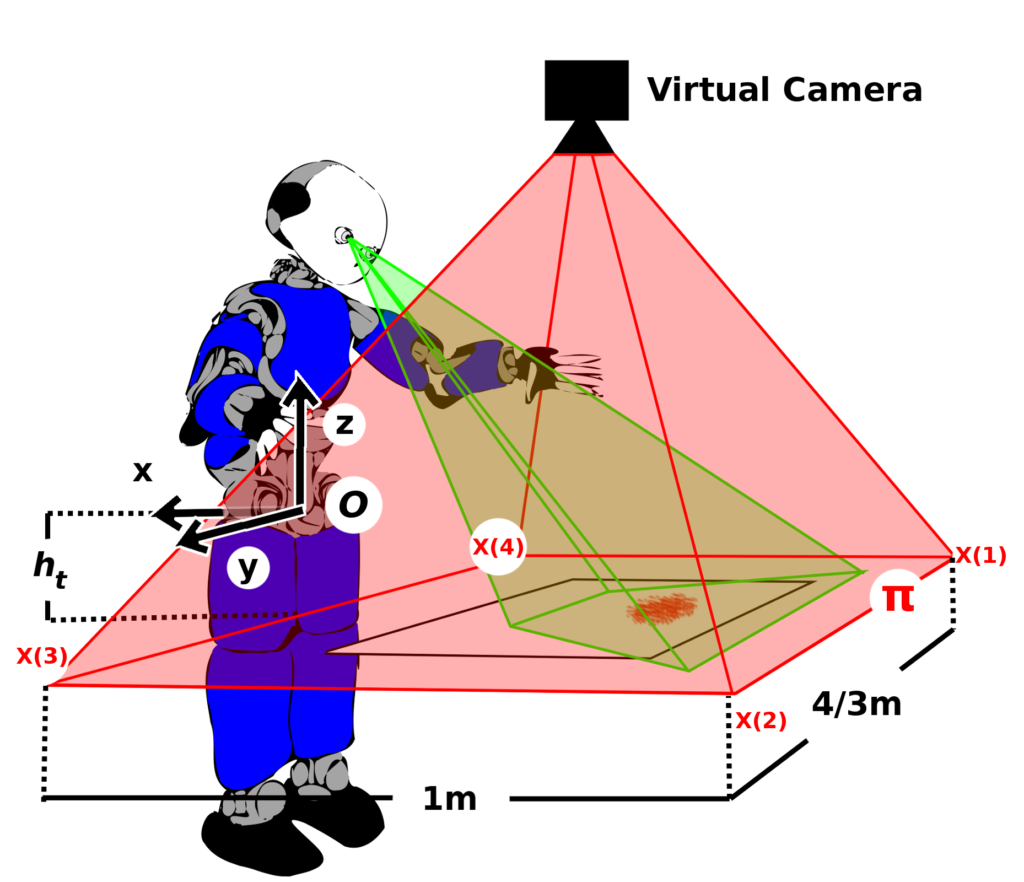
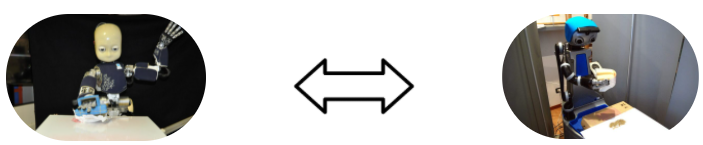
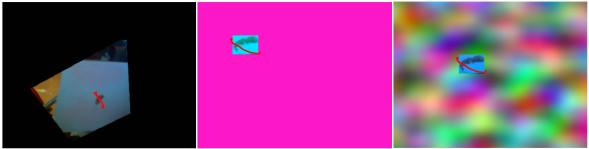
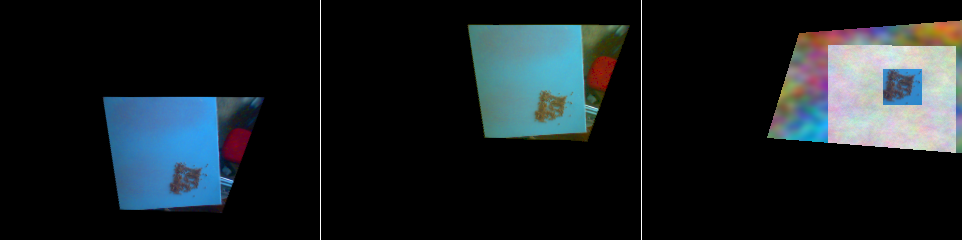
CAD models (PDMS molds) for tactile sensors of Vizzy humanoid robot,
September 2016.
Download link: tactile_sensors_pdms_molds.zip.
Shapes 2018: Images of spheres, cylinder and boxes for shape detection,
July 2018.
This dataset contains 250 images with several household objects, which belong to one of 3 categories: cylinder, box or sphere.
Each image is annotated with bounding boxes and respective class labels.
Technical details are given in the file README.md. For more information, please contact jborrego at isr.tecnico.ulisboa.pt.
See also this GitHub repository.
Download link: tar.gz (73 MB), md5 checksum.
If you use this dataset in your work, please cite the following publication(s):
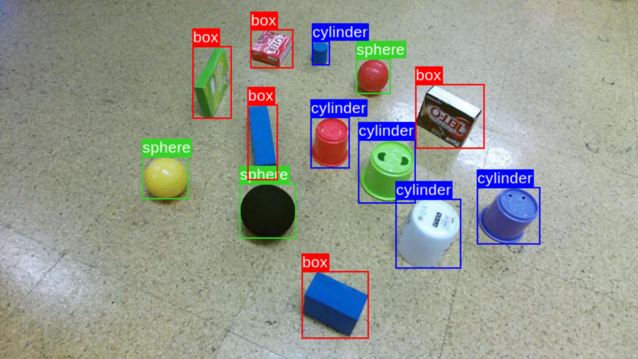
The impact of domain randomization on object detection: a case study on parametric shapes and synthetic textures, A. Dehban, J. Borrego, R. Figueiredo, P. Moreno, A. Bernardino, J. Santos-Victor, in IEEE/RSJ International Conference on Intelligent Robots and Systems (IROS) , 2019. PDF